Projects
Cold Start Problem in Adaptive Fact Learning
Adaptive learning systems need some time to figure out how difficult individual items are for individual learners. During this 'cold start', the system can be poorly adapted to the learner. Given previously collected learning data from other learners/materials, we can try to predict individual difficulties, so that the system is better attuned to the learner from the start. This paper shows that mitigating the cold start in this way can improve learning outcomes.
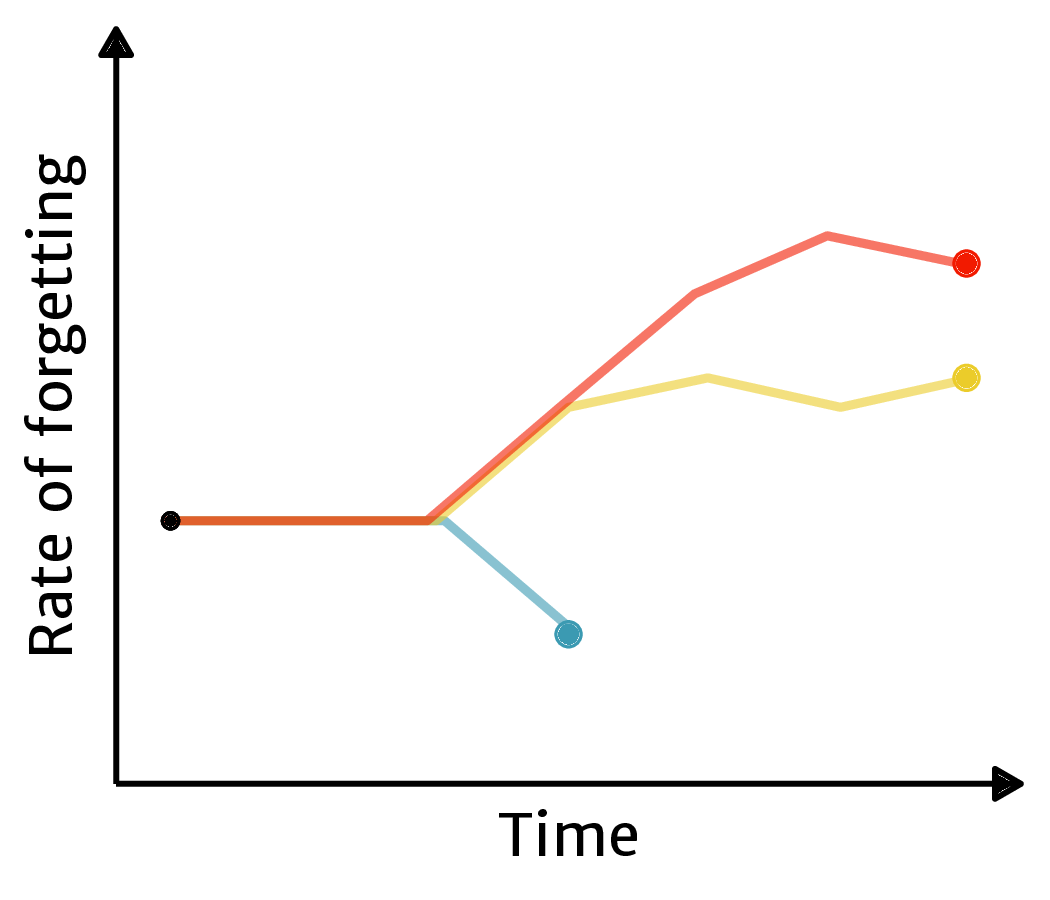
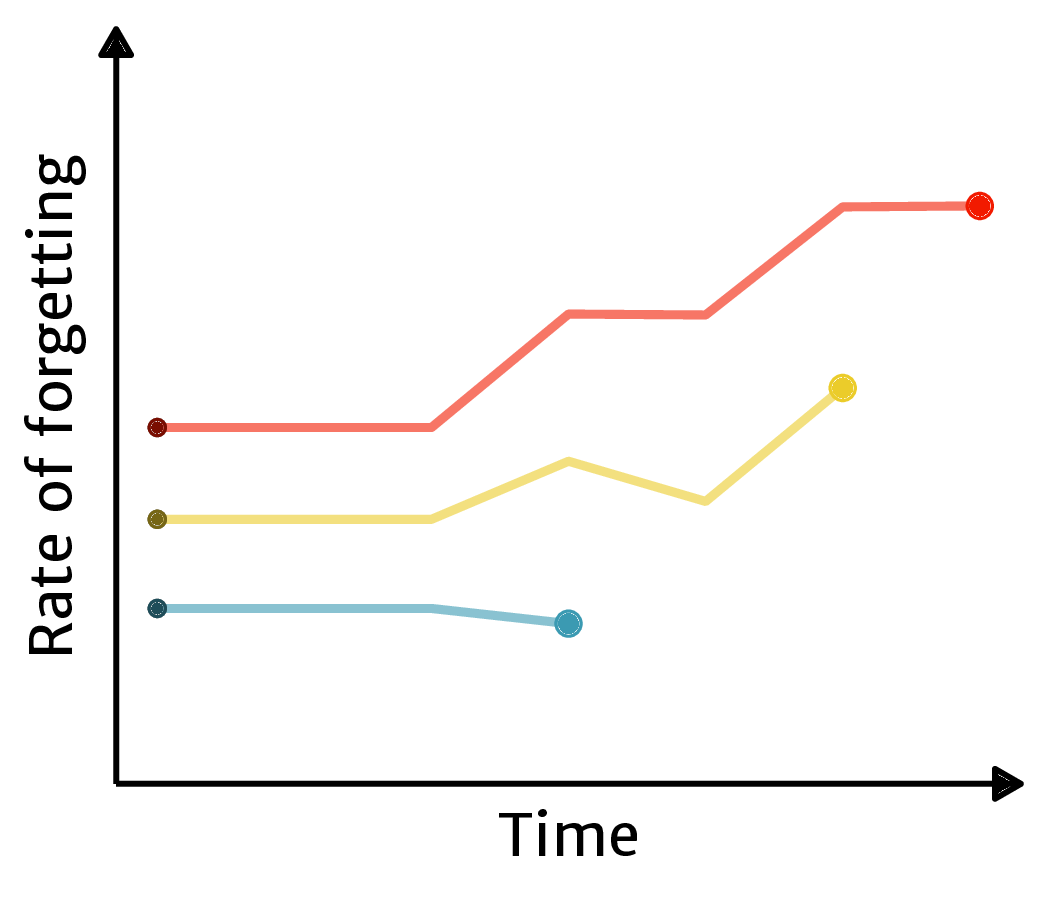
Modelling Cognitive Factors that Contribute to Retrieval Task Performance
Using evidence accumulator methods, we can explicitly model the effects of motor speed and response caution on response time and accuracy in memory retrieval tasks. By accounting for these other cognitive factors we can obtain a more accurate estimate of the thing we're most interested in: memory strength. This paper shows an example where a change in non-memory parameters explains a change in performance on a retrieval task.
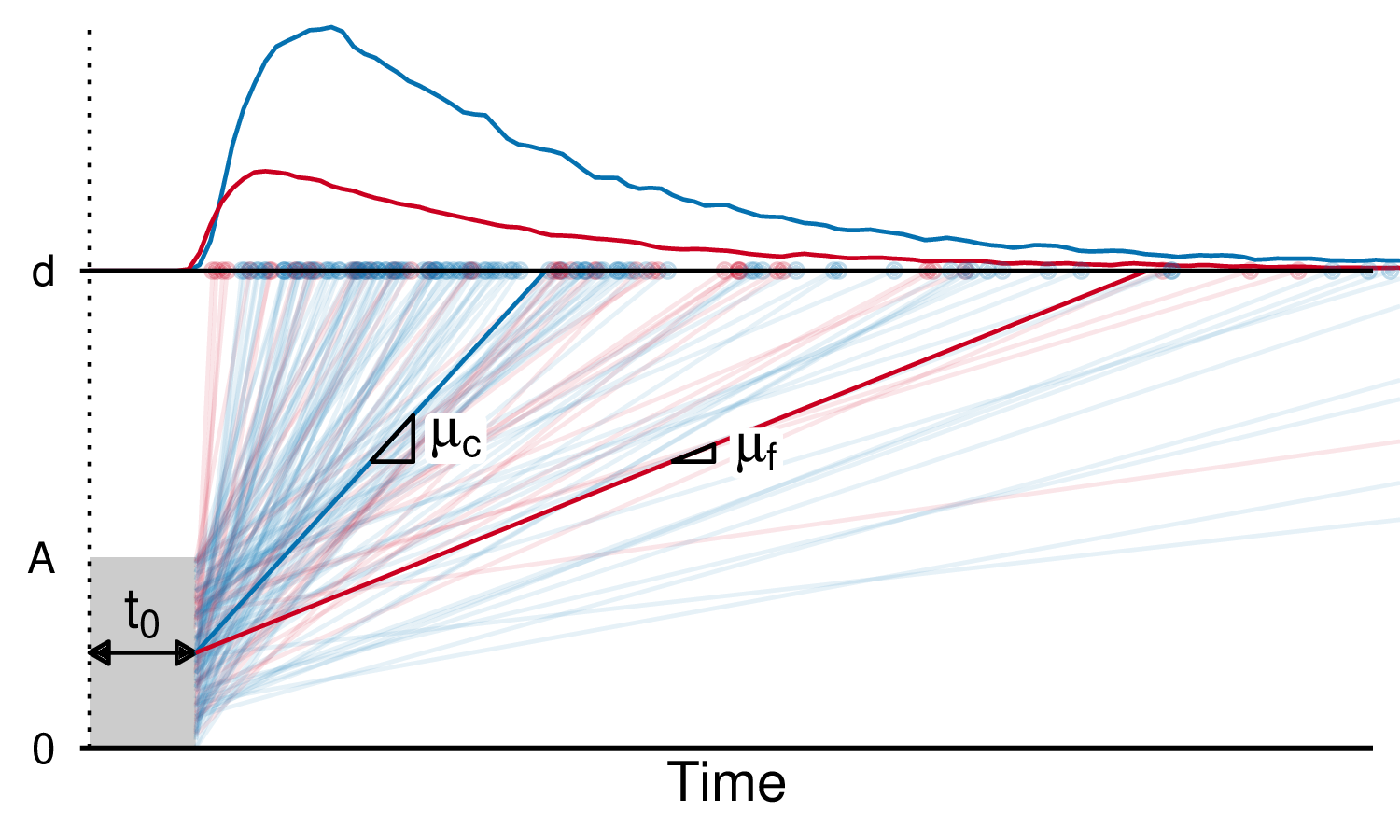
Using Adaptive Fact Learning in Education
Adaptive learning may work well in the lab, but how does it fare in the real world? By collecting data from high school and university students using adaptive learning as part of their curriculum, we can explore the value of model-based adaptive learning under uncontrolled conditions. Does it improve learning? Can we encourage effective study strategies? How can we give students and their teachers more insight into the learning progress? This paper shows that the individual differences in memory performance estimated by the adaptive model during a university course are predictive of exam performance. In this paper, we analyse how adaptive learning of foreign-language vocabulary was affected by the COVID-19 pandemic, based on a very large sample (133K students; 115M trials) of Dutch secondary school students.
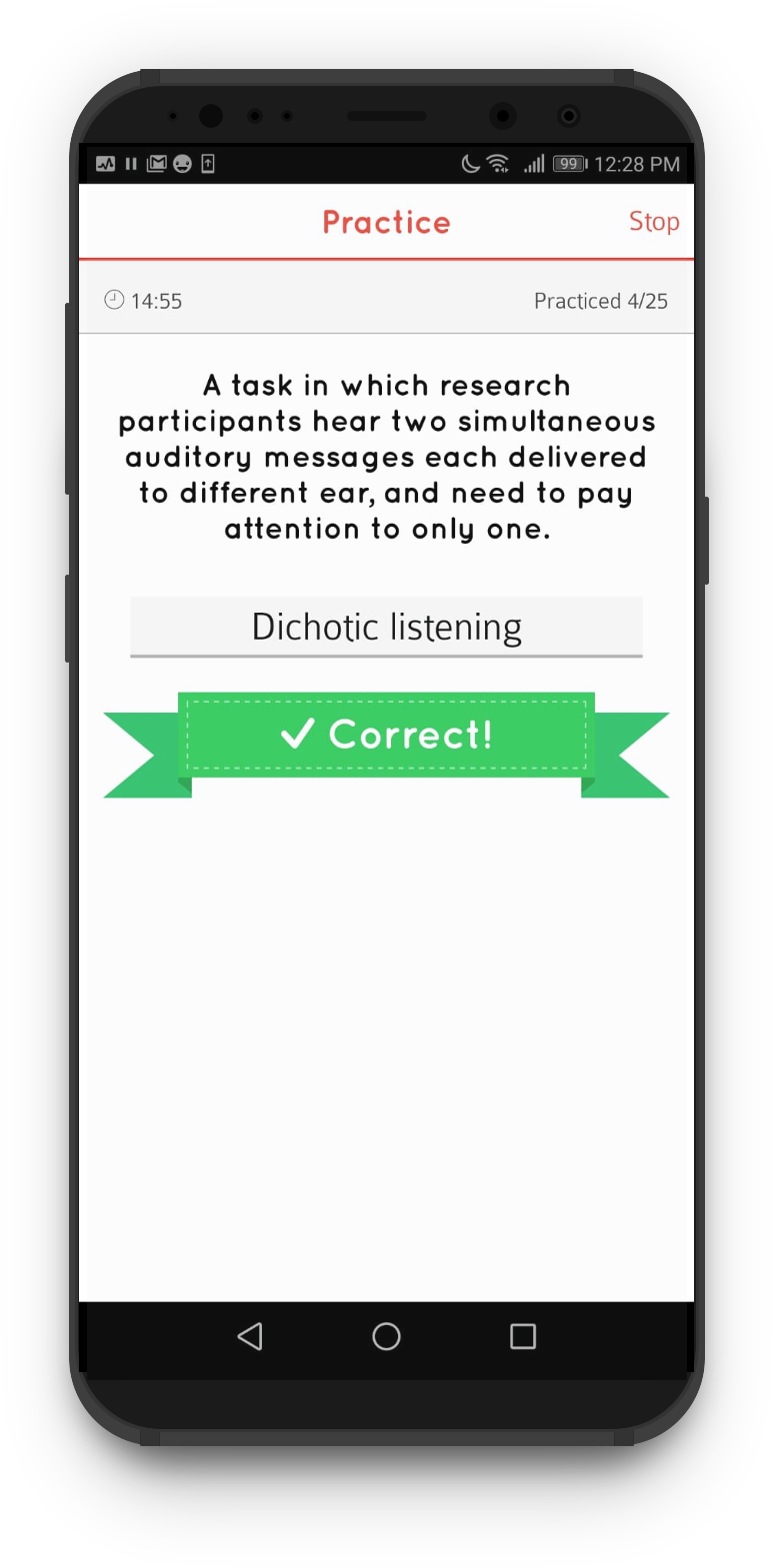